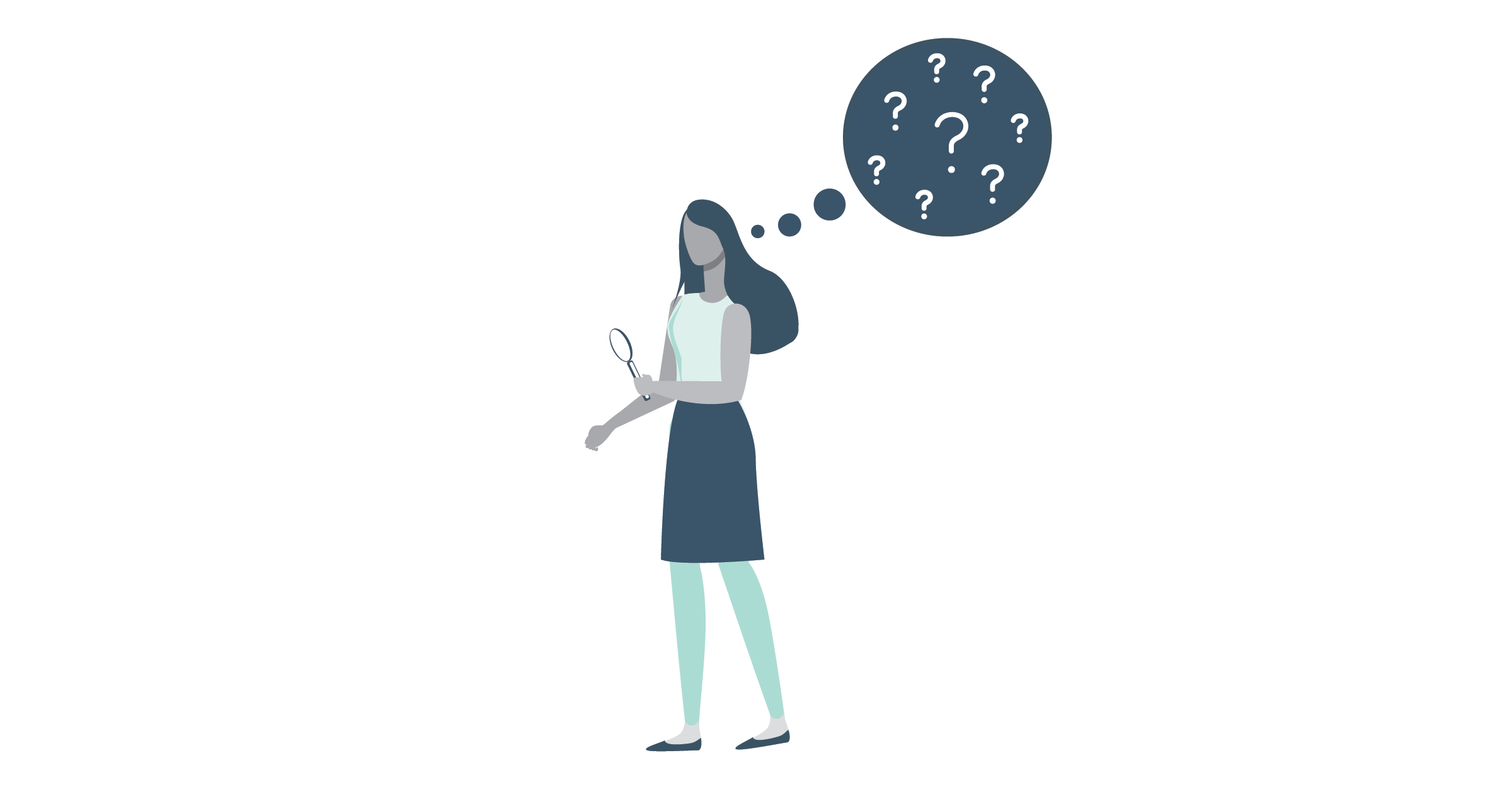
Diabetes care providers have an opportunity to improve diabetes care and avoid potential patient vision loss for the 422 million people living with diabetes around the world through improved diabetic eye exam quality measure performance.¹,² Artificial intelligence (AI) diagnostic systems can autonomously diagnose patients for diabetic retinopathy (including macular edema), allowing medical professionals to offer earlier intervention to the 37 million people with diabetes and more than 60,000 people nationally who go blind from diabetic retinopathy complications.³,⁴
It is important to perform your due diligence when deciding on such a system for your facility. We’ve made it easy with eight questions to ask when reviewing an autonomous AI system for diabetic eye disease.
1. Was the clinical trial published in a peer–reviewed publication?
Clinical trial results should be peer-reviewed by experts in the same field to prevent flawed research from being published. Having results externally validated by other medically relevant experts in the field can confirm results from a proven scientific basis. It shows the study author is willing and open to disagreement and dialogue and committed to transparency. This is an important consideration when deciding on any autonomous AI system.
Another equally important aspect of a system’s clinical trial is the type of trial design that is used. The highest level of validation comes from a prospective clinical trial which requires the establishment of predetermined study endpoints that must be achieved for the trial to be considered a success.
2. Did the device go through FDA De Novo process, or did it go through the 510k process?
Some AI systems are the first of their kind to be marketed and, therefore, must go through the De Novo clearance pathway with FDA. De Novo clearance is a robust and rigorous process that requires a device to be shown as safe, effective, and equitable. This is in contrast to the 510K clearance process which requires a device to demonstrate equivalence to another existing, legally marketed device. Once a system has successfully gone through the De Novo clearance process, that system will then be used as the foundation all future 510K clearances are built upon. De Novo authorization has the highest burden of proof requirement.
3. Was an OCT reference standard used?
Autonomous AI diagnostic results must be compared to the highest possible reference standard that correlates to patient outcomes as the reference to ensure the safety, efficacy, and equity of the autonomous AI. For autonomous AI diagnostic systems that diagnose patients for diabetic retinopathy (including macular edema), the highest possible reference standard is an independent reading center such as the University of Wisconsin’s Fundus Photography Reading Center, which created the gold standard for diabetic retinopathy treatment along with the National Eye Institute. Using a respected, centralized source and multiple imaging modalities, such as wide-field fundus photography and ocular coherence tomography (OCT) as the reference standard for a clinical trial allows several image types to be studied and classified by highly trained readers that have no stake in the new technology or outputs prior to their independent classifications. Knowing the reference standard used in a trial can help you better understand the level of validation a system has received.
4. Was the clinical trial run exclusively in primary care or was an ophthalmology setting also used?⁵,⁶
It is important to understand the setting in which clinical trial data was captured as it can have an impact on system accuracy and integration. If the intended clinical workflow for a system is implementation into a primary care office with a novice camera operator and a highly diverse population, the clinical trial design needs to mimic those conditions to truly be an accurate representation of the systems results. If the trial was executed in an exclusively ophthalmic setting the results would not be accurate to novice operators capturing images in point-of-care settings. Similarly, if all the test subjects were the same age or gender it would be impossible to verify how the results would differ in a more diverse population.
5. Were novice camera operators used in the trial, or were some of them ophthalmic technicians?
It is important to ask if a study was performed with ophthalmic technicians, or if novice camera operators also participated to help clarify the study results and inform how the system will integrate into your facility. Ophthalmic technicians have advanced training and understanding of ophthalmic imaging systems which could skew study results if the intended workflow is not in an ophthalmic setting. AI diagnostic systems should be easy to operate for those in the primary care or even pharmacy settings with minimal training. Easy integration into existing staff workflow is part of what makes it possible to offer diagnostic results at the point-of-care with no need for specialist overread.
6. Does the system actively improve access?
People living with diabetes may experience limited access to specialty care, an issue that was exacerbated by COVID19 as access to examinations with eye care specialists were severely limited. The right AI diagnostic system should mitigate exam access issues by supporting primary care to implement systems at the point-of-care while increasing actionable eye care referrals. Johns Creek Primary Care recently doubled their referable cases of diabetic retinopathy after adopting Digital Diagnostics’ LumineticsCore™ (formerly known as IDx-DR), and increased compliance rates with diabetic retinal exams from 16% to 51% based on year over year data comparison.
7. If patients were removed from the statistical analysis, what was the reason?
This question should give insight into the validity and quality of the clinical analysis. Missing data is a frequent research obstacle. However, it can have a large impact on the data. An absence of data can decrease statistical power and increase bias. When considering an AI diagnostic system, you should not only have access to the research, also to the data behind it. If patients are removed from the statistical analysis, the reasons those patients were removed should be stated to ensure they were omitted for valid reasons that did not increase bias.
8. Does the creator of the AI diagnostics system assume liability?
For a traditional diagnosis made by a medical professional, the liability for the diagnostic outcome rests with the diagnosing physician. For autonomous AI diagnostic systems where the diagnosis is made by the computer and operators are not required to have specialty training, the liability should rest with the developer of the AI system.⁷
These eight questions can help identify the ideal autonomous AI diagnostic system for your facility. After considering all of the details, adopting such a system should increase access to care for patients, be easy to implement into existing workflows, be simple enough to use with minimal training, and help physicians close care gaps. They should also be backed by proper research and rigorous FDA processes.
Looking for more on this subject? Read How to Choose the Reference Standard for an AI Clinical Trial.
LumineticsCore (formerly known as IDx-DR) is for care providers to help address disparities and improve equity in care as part of the diabetic eye exam delivery model. LumineticsCore (formerly known as IDx-DR) outputs can direct more actionable referrals, identifying patients with diabetes, not already under the care of an eye care provider, who are most likely to need timely vision saving interventions. Learn more about its impacts to closing care gaps and preventing blindness.
References
- Diabetes. (n.d.). Retrieved March 24, 2021, from https://www.who.int/health-topics/diabetes#tab=tab_1National Eye Institute (2019). Diabetic retinopathy data and statistics. Accessed September 1, 2020. https://www.nei.nih.gov/learn-about-eye-health/resources-for-health-educators/eye-health-data-and-statistics/diabetic-retinopathy-data-and-statistics
- Economic Studies|Vision Health Initiative (VHI). (2017, April 11). Accessed September 01, 2020. https://www.cdc.gov/visionhealth/projects/economic_studies.htm
- Varma, R., et al (2016). Visual Impairment and Blindness in Adults in the United States. JAMA Ophthalmology, 134(7), 802. doi:10.1001/jamaophthalmol.2016.1284
- CDC. (2019). National Diabetes Statistics Report. Center for Disease Control and Prevention. https://www.cdc.gov/diabetes/data/statistics-report/index.html
- https://www.ama-assn.org/system/files/2019-08/ai-2018-board-policy-summary.pdf
- Abràmoff, M. D., Cunningham, B., Patel, B., Eydelman, M. B., Leng, T., Sakamoto, T., Blodi, B., Grenon, S. M., Wolf, R. M., Manrai, A. K., Ko, J. M., Chiang, M. F., & Char, D. (2021). Foundational Considerations for Artificial Intelligence Using Ophthalmic Images. Ophthalmology, 0(0). https://doi.org/10.1016/j.ophtha.2021.08.023
- Health care AI must boost the quadruple aim to move forward. (n.d.). American Medical Association. Retrieved March 28, 2022, from https://www.ama-assn.org/practice-management/digital/health-care-ai-must-boost-quadruple-aim-move-forward