What is a reference standard?
When validating a new AI diagnostic technology, it is essential to have a high-quality reference standard or “truth” against which the new technology can be assessed. In a clinical trial of a moderate to high risk autonomous AI system, an AI’s diagnostic (or therapeutic) results must be compared to a rigorous standard that correlates to patient outcomes as the reference to get an accurate assessment of the safety, efficacy, and equity of the AI.
However, not all reference standards are created equal, and some are less objective than others. The table below outlines various components involved in determining the credibility of a reference standard being used to measure clinical trial outcomes.
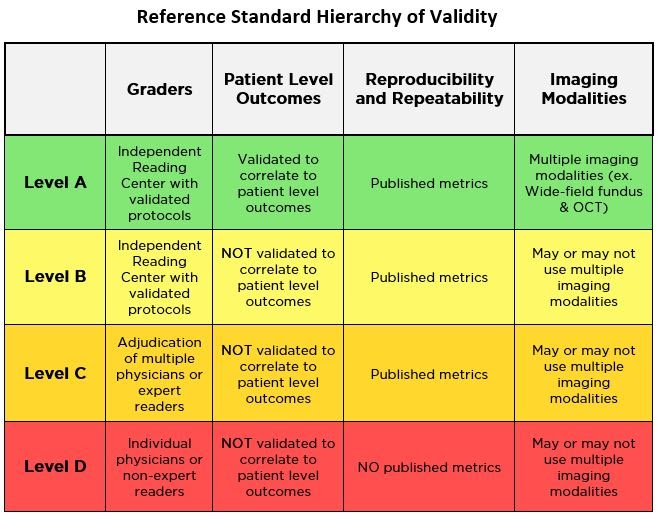
To understand this further, let’s examine LumineticsCore® (formerly known as IDx-DR), the first FDA De Novo cleared autonomous AI diagnostic system, as a case study for following a robust ‘Level A’ reference standard that allows for the objective assessment of the safety, efficacy, and equity of an AI.
Deciding the reference standard
During validation of an AI diagnostic system there needs to be a consistently high standard for the data used during assessment. If the images acquired for validation have been evaluated by a single clinician or an adjudication of clinicians for clinical purposes, they may not correspond to real world clinical outcomes. When this happens, the AI is tested against a specific clinician or group’s assessments, not a generally applicable patient level outcome.
This can have an effect on the AI’s safety, efficacy, and equity when compared to a true clinical outcome. A more objective reference standard is the use of a reading center, which has expert graders and quality controls in place to limit observer variation when assessing images. Even better is if the reading center being used was responsible for developing the gold standard for diagnosis and treatment of the disease the AI is diagnosing.
In the case of LumineticsCore® (formerly known as IDx-DR), the reference standard used for its clinical trial was the University of Wisconsin’s Fundus Photography Reading Center [i], which was responsible for the creation of the gold standard for treatment of diabetic retinopathy in conjunction with the National Eye Institute (NEI) [ii]. In addition, the center’s founder Matthew Davis, MD was a collaborator in the development of the classification of diabetic retinopathy (ETDRS) [iii] that has been used in clinical research for decades and is the current scale used to diagnose diabetic retinopathy.
The ETDRS scale serves as a surrogate to conducting a study that tracks the real time progression of diabetic retinopathy. Conducting a new outcome-based study for AI validation would require the disease state to go untreated for the purpose of observing the progression of symptoms, eventually leading to advanced complications and possible visual loss for patients in the study. Using the ETDRS grading truth from the original outcomes study center gives the best alignment to outcomes while remaining ethical and safeguarding patient safety.
Using a respected, centralized source, such as the Fundus Photography Reading Center, as the reference standard for a clinical trial allows the images to be studied and classified by highly trained readers that have no stake in the new technology or outputs prior to their independent classifications.
Using a centralized reference standard, like the Fundus Photography Reading Center, mitigates the potential for bias in the results and ensures a transparent and truthful evaluation of the new technology.
Without a centralized reference standard it becomes far too easy for entities to set their own reference standards that could make their product look overly favorable. For a new technology to be completely trustworthy, the methods used to test its efficacy must be as robust as possible.
If the reference standard is not hosted by a neutral third party, it becomes hard to verify whether bias was present in the trial results. This allows for the possibility that one or more of the readers responsible for the reference standard may have drifted from an established patient level outcome.
Applying the Clinical Gold Standard
To rigorously validate an AI’s safety and efficacy at diagnosing a specific disease state, the reference standard used should be based on the clinical gold standard (Level A) – the best available diagnostic test(s) for determining whether a patient does or does not have a disease or condition.
In the case of diabetic retinopathy and diabetic macular edema, the current gold standard clinical evaluation includes 3D optical coherence tomography (OCT) and wide-field stereo fundus imaging.
To stay in alignment with the clinical gold standard, the LumineticsCore® (formerly known as IDx-DR) clinical trial also incorporated OCT and wide-field stereo fundus imaging. This means the graders at the reading center had access to significantly more information than the LumineticsCore® (formerly known as IDx-DR) system, which created a diagnostic result based off two-field fundus photography.
Despite LumineticsCore® (formerly known as IDx-DR) having access to less data than the graders at the reading center, the system was able to achieve high diagnostic performance that produced consistent, reliable results which were in line with the outputs of the highest-level reference standard.
While it would have been possible to use a reference standard that only included fundus photography to match the AI’s inputs, testing against the Level A gold standard allowed for more rigorous assessment and validation of LumineticsCore® (formerly known as IDx-DR).
Why the reference standard matters
Any new medical technology or innovation would benefit from being checked against a patient level outcome-based reference standard to ensure that the advancements being made are in line with current clinical practices and offer real world benefit. In the case of LumineticsCore® (formerly known as IDx-DR), using multiple clinically relevant imaging modalities and seeking the reference standard from an independent source like The Fundus Photography Reading Center helped prioritize a patient level outcome.
In addition, using a reference standard with clearly defined parameters allowed for data outcomes that were verifiable, repeatable and reproducible to avoid and correct for bias whenever possible.
Because AI for medical diagnosis is a relatively new field, it is imperative that validation of new technologies is regulated and verified so that patient safety is never compromised. As more clinical trials are conducted on emerging medical technology, understanding the reference standards that are applied to each will help cultivate a better understanding of clinical trial data and the patient outcomes that may follow.
References
[i] Fundus Photograph Reading Center[Internet]. UW DOVS. Available from: https://www.ophth.wisc.edu/research/fprc/
[ii] National Eye Institute [Internet].National Eye Institute. U.S. Department of Health and Human Services; Available from: https://nei.nih.gov/
[iii] Early Treatment Diabetic Retinopathy Study (ETDRS) – Full Text View [Internet]. Full Text View – ClinicalTrials.gov. Available from: https://clinicaltrials.gov/ct2/show/NCT00000151